TensorFlow 1.x 卷积等操作的高级封装
本文于 1244 天之前发表,文中内容可能已经过时。
前一段时间写了一些程序,自己搭建卷积神经网络的时候需要用到不少tensorflow的自带低级封装还有一些常用的读取操作等,虽然已经有了keras等高级封装可以直接调用,但还是想自己写一写。
tensorflow版本:1.14
1. 卷积函数
1 | def weight_variable(shape): |
2. 池化函数
1 | def max_pool(x): |
3. 读取图片顺便加标签
文件夹结构(假设目录下面有17个文件夹,每个文件夹里面有若干对应种类的图片):
1
2
3
4
5
6
7
8
9
10
./
–./dogs
–./cats
–./tigers
…读取的图片压缩为32323的尺寸,便于神经网络的快速迭代
1 | # 调用skimage的函数 |
4. 神经网络模型的建立
下面的示例结构为:3层卷积+2层全连接
图片的输入x_images的shape是32323
1 | def create(x_images,keep_prob):#x_images的shape是32,32,3 |
赏
支付宝打赏
微信打赏
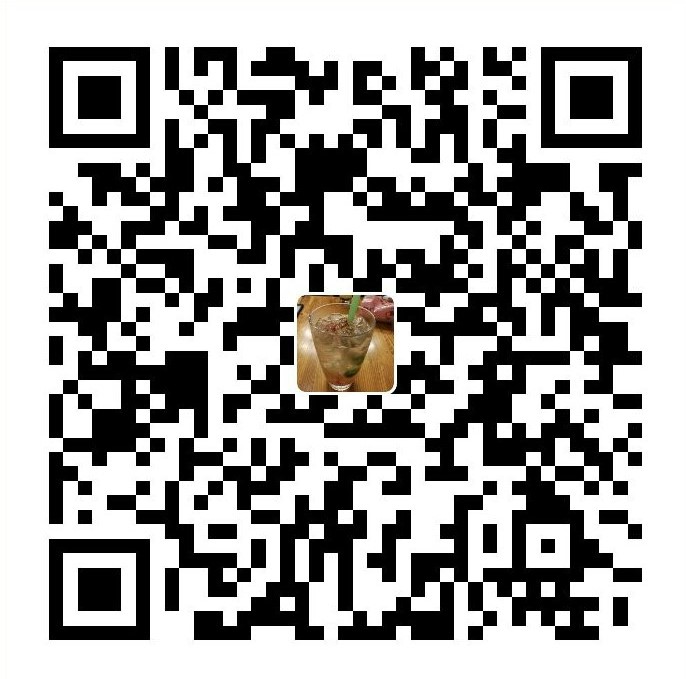
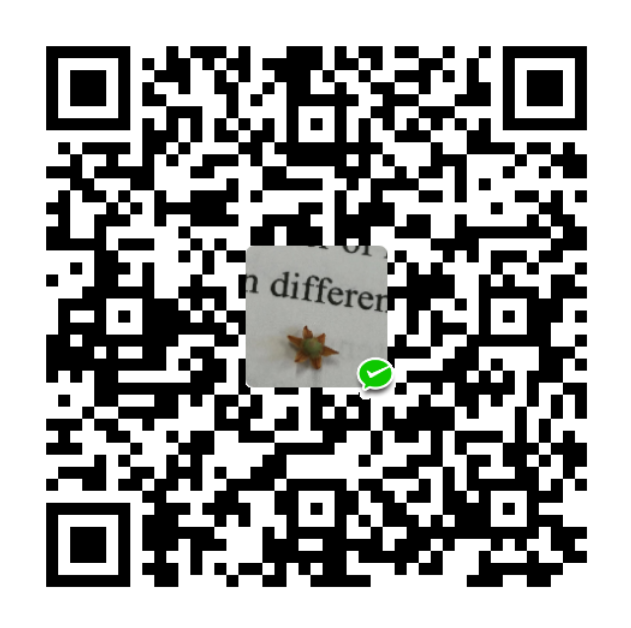
赞赏是不耍流氓的鼓励